It is not uncommon that knowledge about relevant information for a product is held in different places, in different formats and by different people within a company. Translations are scattered as text files in the translation office, product photos at an agency, and master data in Excel spreadsheets or outdated data sheets. The quantity of files containing technical and commercial data relevant to a product is large. Product data means all such information that describes products. Depending on the industry and the user, this can be information in different formats such as images, text or numbers. Without a uniform system, the consolidation is error-prone, time-consuming and duplications are difficult to avoid. This is an additional expense that can be avoided.
Good product data and its management are essential for success in online retailing. The requirements surrounding product data are high and have increased even more due to shorter product life cycles. All touchpoints must be supplied with information throughout the entire customer journey. Here, customer journey means the customer itinerary within a purchasing process, which varies from individual to individual (see Verticalization in e-commerce). The provided product data play a major role in the shopping experience in the onlineshop and ultimately in the customer's purchase decision. The quality of product data thus becomes a prerequisite for the success of a company in e-commerce. Product data become the signboard in the shop window for an online store. It replaces the face-to-face conversation in the store and must ideally demonstrate the expertise that a product seller has. Does the spare part fit my device? Does it contain allergens? Is the lamp waterproof? Is there a series or second volume for the book? Depending on the perspective, these are relevant information that can be criteria for customer decisions and should be mapped in high-quality product data.
Product information management systems are used to manage and prepare product data. These bundle, check and refine product data and can then output it collectively to channels.
Regardless of what information is played into a PIM system, the main requirement of such a system is to create high-quality product data sets.
When data can be classified as high quality depends entirely on its purpose. This purpose can also be described as "fitness for use" - in the sense of usability. This can indicate the degree of effectiveness, efficiency and satisfaction with which a goal is achieved. (cf. Helmis/Holmann 2009) In colloquial language, the term user or operator friendliness is more common. How do product data become user-friendly? And which criteria can be applied?
The American scientists Richard Y. Wang and Diane M. Strong developed a conceptual framework for data quality already in 1996. They name 15 quality characteristics and assign them to four data quality classes.
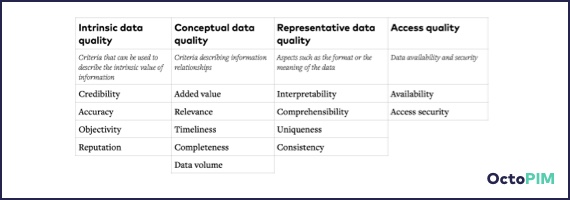
The data quality criteria worked out by Wang/Strong are applied or fulfilled in the rarest cases all at the same time. In accordance with the above-mentioned usability, the selection of the criteria must be made according to the meaningfulness in the respective case.
In the following, possible improvements in product data quality are examined in more detail using the concrete case of three data sets for a fictitious item of clothing.
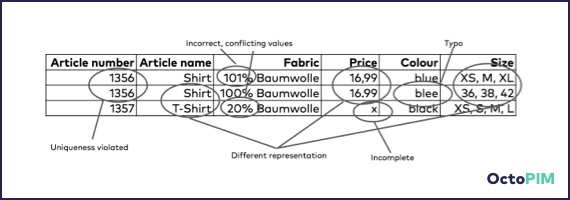
Criteria: Completeness
By definition, a set of data is complete when all defined attributes (properties) - in our example: article number, article name, material, price, color, size - are filled in. If information is missing, such as the price in the last data record, the PIM system draws attention to this in the dashboard so that this error can be corrected. Adding rules, and their severities and parameters define wheter and when information needs to be fillded in.
Criteria: Consistency
In the attribute column for price, the two data sets are not displayed in the same way and are therefore not structured in a uniform manner. Even if it is clear that both representations can be possible representatives of a price, they violate the quality criterion of consistency. A PIM system prevents these errors by defining which characters are allowed in the respective field.
Criteria: Accurancy
Data sets are accurate - consistent - if they do not show any inconsistencies within themselves and with others. This is one, if not the basic requirement for product data. From a company's point of view, inconsistent data produces additional work. This in turn leads to increased costs due to the necessity to improve errors. From the customer's point of view, inconsistent data leads to dissatisfaction, raises doubts about credibility and often leads to migration to other providers.
Creating and ensuring consistent data is the core business of a PIM system.
Conclusion
Product data is an important component for the design and optimization of a digital shop window. Depending on the use case, adapted criteria help to check and improve the quality of the product data.